Aug 07, 2024
Abstract:As Large Language Models (LLMs) become increasingly sophisticated and ubiquitous in natural language processing (NLP) applications, ensuring their robustness, trustworthiness, and alignment with human values has become a critical challenge. This paper presents a novel framework for contextual grounding in textual models, with a particular emphasis on the Context Representation stage. Our approach aims to enhance the reliability and ethical alignment of these models through a comprehensive, context-aware methodology. By explicitly capturing and representing relevant situational, cultural, and ethical contexts in a machine-readable format, we lay the foundation for anchoring a model's behavior within these contexts. Our approach leverages techniques from knowledge representation and reasoning, such as ontologies, semantic web technologies, and logic-based formalisms. We evaluate our framework on real-world textual datasets, demonstrating its effectiveness in improving model performance, fairness, and alignment with human expectations, while maintaining high accuracy. Furthermore, we discuss the other key components of the framework, including context-aware encoding, context-aware learning, interpretability and explainability, and continuous monitoring and adaptation. This research contributes to the growing body of work on responsible AI, offering a practical approach to developing more reliable, trustworthy, and ethically-aligned language models. Our findings have significant implications for the deployment of LLMs in sensitive domains such as healthcare, legal systems, and social services, where contextual understanding is paramount.
* World Journal of Advanced Engineering Technology and Sciences,
2023, 10(2), 283-296
* 14 pages
Via
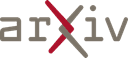